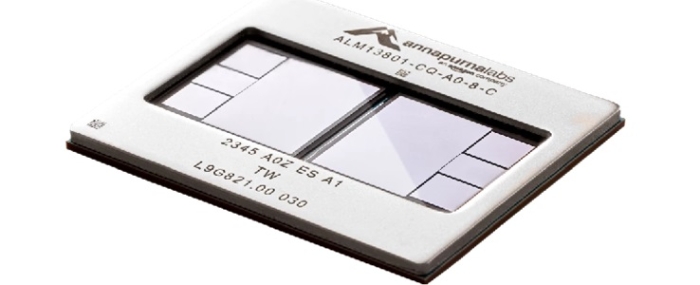
Amazon’s Trainium3 chip to be released by end of 2025
The new chip is expected to be up to twice as fast as its predecessor, Trainium2, and offer 40% better energy efficiency.
Amazon’s new self-developed chip, Trainium3, which will be fabricated with 3nm, is set to be released by the end of 2025.
“Trainium3-powered UltraServers are expected to be 4x more performant than Trn2 UltraServers, allowing customers to iterate even faster when building models and deliver superior real-time performance when deploying them,” Amazon Web Services (AWS) said in a media release.
The new chip is expected to be up to twice as fast as its predecessor, Trainium2, and offer 40% better energy efficiency.
Amazon has also unveiled new data center servers featuring its proprietary Trainium2 AI chips. Apple is among the new clients that are adopting Trainium2 chips.
AWS’s newly unveiled Trn2 UltraServers are being seen as a competitor to NVIDIA’s flagship servers, which feature 72 of its cutting-edge Blackwell chips, according to a Reuters report.
The Trn2 UltraServers enable customers to train and deploy today’s latest AI models as well as future large language models (LLM) and foundation models (FM), the company said.
The Trn2 UltraServers are a completely new EC2 offering featuring 64 interconnected Trainium2 chips, using ultra-fast NeuronLink interconnect, to scale up to 83.2 peak petaflops of compute — quadrupling the compute, memory, and networking of a single instance — which makes it possible to train and deploy the world’s largest models, AWS said.
“Trainium2 is purpose-built to support the largest, most cutting-edge generative AI workloads, for both training and inference, and to deliver the best price performance on AWS,” said David Brown, vice president of Compute and Networking at AWS. “With models approaching trillions of parameters, we understand customers also need a novel approach to train and run these massive workloads. New Trn2 UltraServers offer the fastest training and inference performance on AWS and help organizations of all sizes to train and deploy the world’s largest models faster and at a lower cost.”